Orca's self-tuning, memory-driven platform
Orca's ML Ops platform allows you to build predictive AIs steered by interchangeable, auditable memories. Because Orca-based models use specialized external memory for every inference, your predictive AIs now respond to updated information - without requiring retraining. Your model can even select different memories based on pre-defined signals every time your AI gets used.
Step 1: Create a memoryset
Orca automatically transforms your data into a "memoryset," which allows Orca's foundational predictive AIs to understand and use your unique data during inference. Orca's data-management co-pilot uses this understanding to diagnose and immediately correct mislabeled, missing or unneeded data, ensuring you quickly and confidently know your AI's memory aligns to the desired task.

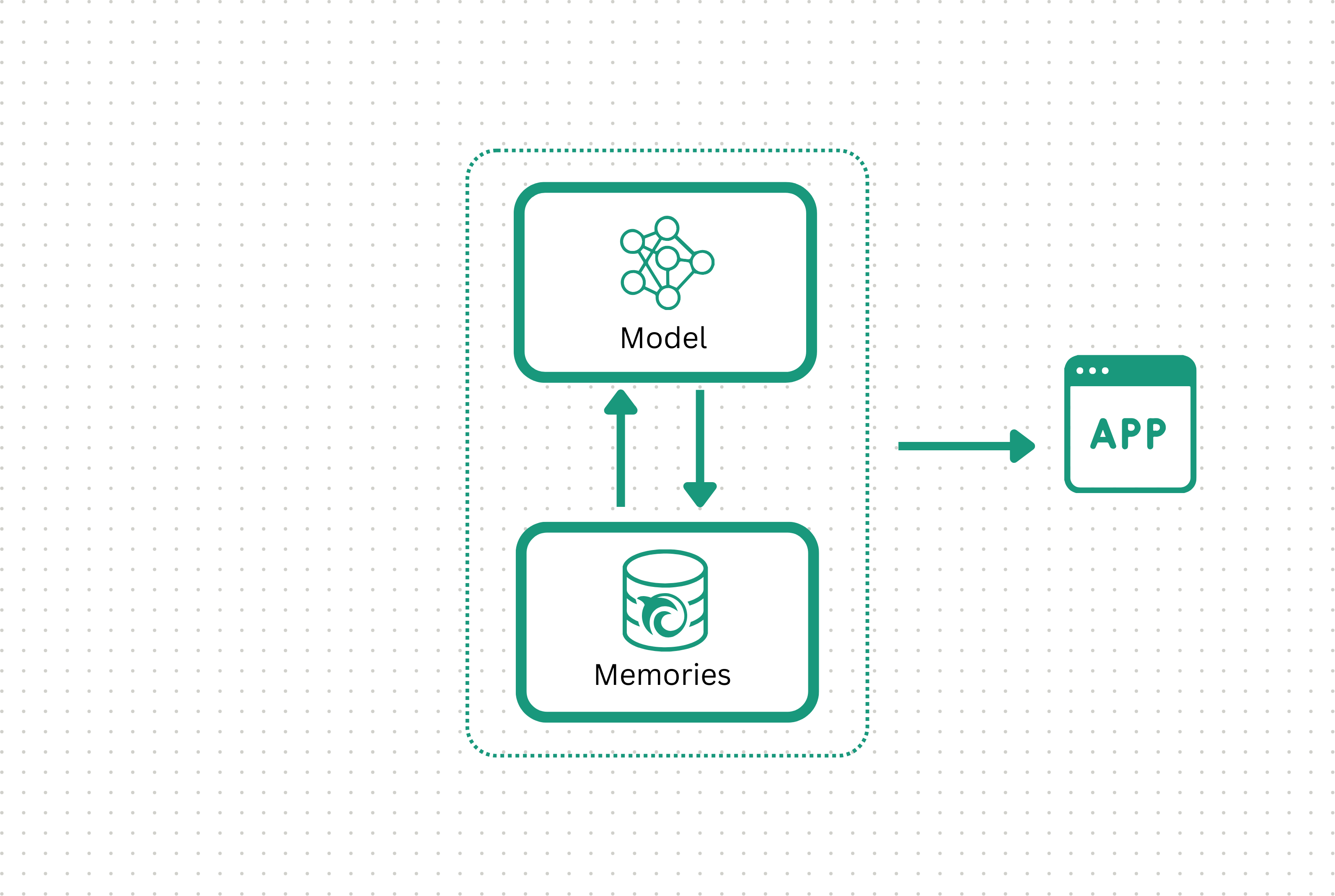
Step 2: Reach optimal accuracy
With a well curated memory, your AI quickly reaches the level of accuracy you demand. Once you are satisfied with its performance, you can promote this AI to production.
Orca can host your AI and data or you can leverage Orca's tools while keeping sensitive data within your environment.
Step 3: Correct and improve instantly
After an AI is in production, Orca actively connects and tracks business outcomes back to the specific memories your AI used for that inference run, so diagnosis can happen immediately. Then, remediation simply requires minor edits to the relevant memories so the model can learn new or changed information in near real time.
Orca also proactively detects higher risk areas - times your AI answers correctly, but memory is less robust and the AI has lower confidence. Through this identification, Orca can augment your memoryset to make the AI more reliable in the future.
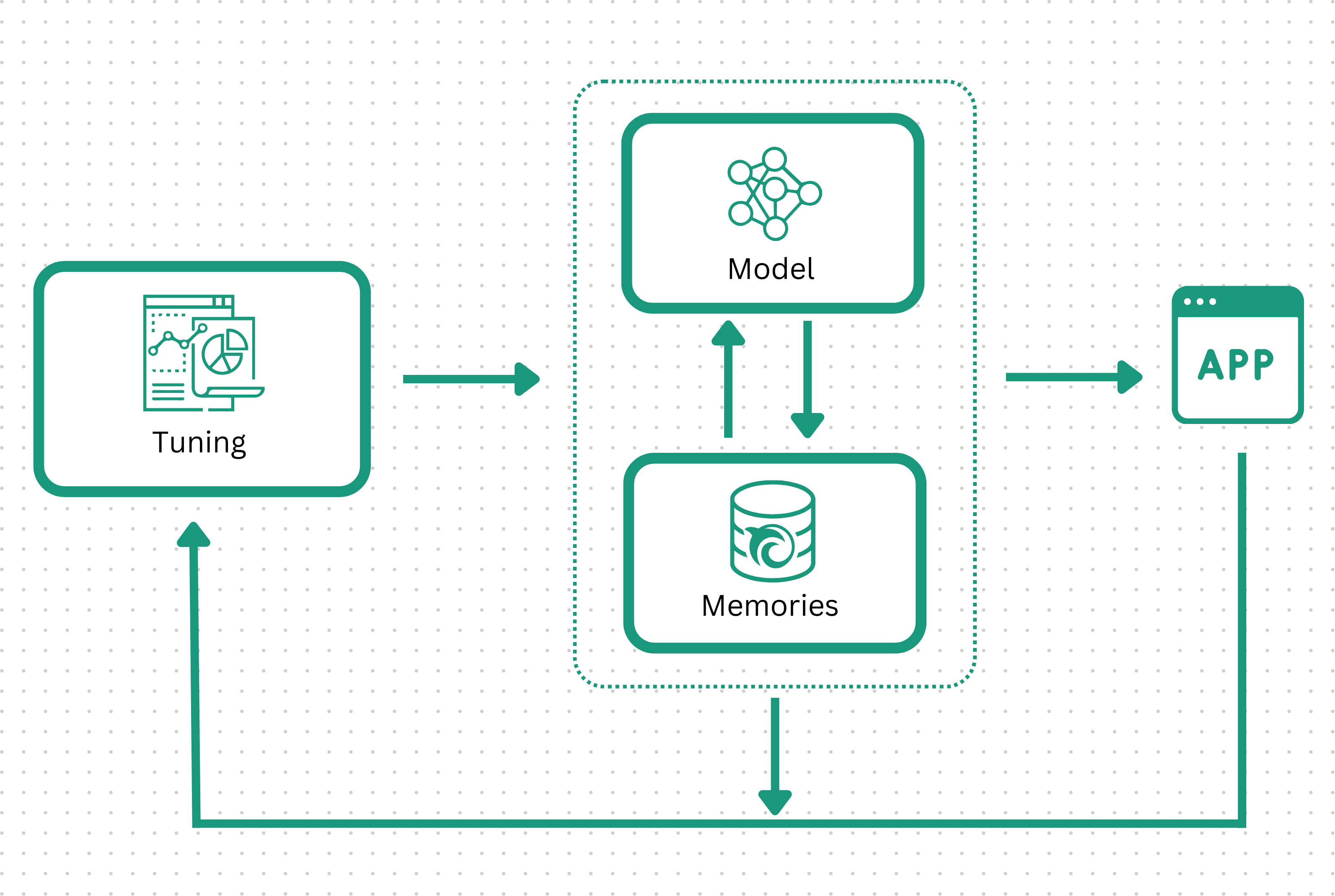

Step 4: Configure datasets for mass customization
The AI's memory-based architecture also unlocks mass customization. Orca models can select different memorysets at inference without any retraining. This allows subtle changes to your data—which are often lost or overfit during retraining—to actively evolve your AI. This powerful property steers AIs to make different predictions for unique populations and situations.
Customizing predictive AI with Orca also avoids the operational headaches incurred by supporting a significant number of unique models. Simply have Orca use external signals to direct the hosted model to select from different external datasets at inference.
Orca builds memory-driven AIs
Orca augments its foundational models with memories. Just like retrieval augmentation with large language models leveraging external context, predictive AIs built with Orca maintain logic and reasoning in the foundation model, but supply “correctness” from an editable, external memory. Even better - Orca’s models explicitly use context, instead of simply relying on poorly understood emergent properties, so you don’t have the risk of hallucinations.
FAQs
Let's dive into the details.
Traditionally, predictive AIs develop their knowledge by memorizing data during a pre-production training phase. Once deployed, the memory does not get updated - to update the memory a retraining or finetuning is required which occurs at intervals constrained by cost, time, and expertise. Orca fundamentally changes this architecture by enabling the base ML model to follow a dynamically updated memory during production. This allows more continuous tuning and training and an inference time “swap out” of memory to enable whole new classes of customization use cases (e.g. customizing the response of a model to individual users).
AIs are notoriously difficult-to-decipher black boxes - you never know what underlying data caused a model to make its prediction. This effect makes retraining, tuning, and debugging an AI inherently challenging to do quickly and confidently.
Because Orca integrates the memory tightly with the model, each model output can be traced to the individual data points that led to the output. This enables auditable, explainable reasoning and confident data labeling/editing.
Orca prioritizes predictive AIs that traditionally memorize training data. We replace the retraining bottleneck with customizable memories, so you can tackle challenges like accuracy degradation, misbehavior, and mass customization without ever taking your model offline. Example models include:
- Text classifiers
- Image classifiers
- Ranking models (including within recommendation systems)
While Orca supports a wide range of predictive use cases, Orca’s unique context memory architecture and ability to overcome the black box effect is most impactful for situations with rapidly evolving data, significant consequences for mistakes, or the need for highly customized predictions (or several of those challenges). Example applications of Orca’s platform include:
- User-specific recommendation systems for ad targeting
- Classifiers powering threat detection for cybersecurity, where any lags for retraining can be rapidly exploited
- Object detection for driver assistance systems that need to adapt to new local environments
- Adaptive recommendations on an e-commerce site that continually adapt, at an individual level, based on each new pageview
- Toxicity moderation in social media that needs to adapt to new slang
- LLM governance systems that need to customize to define if a prompt is on-brand or not for each client’s chatbot
- Fraud detection where adversarial adaptation demands high retraining and tuning frequency
- A/B testing where quickly swapping memory datasets is much faster and more efficient than running multiple entire train-run-inspect cycles
The key difference between Orca and other ML Ops tools is that Orca creates memory-based predictive AI. This architecture unlocks several critical challenges, including:
- Accuracy degradation: Unlike traditional models that rely on memorized training data, Orca’s models leverage specialized external memories that steer the model’s decision making. Because the model proactively uses these memories for each inference run, any information you add to boost accuracy immediately steers the model, even without retraining.
- Misbehavior: The memory architecture allows you to connect the exact memories the model used, the outputs from your AI, and the actual outcome (e.g. the user clicking on the recommended article or an email flagged as spam actually moving to the inbox). This overcomes the limitations imposed by the black-box nature of traditional predictive AIs and allows remediation with minor edits to the relevant memories, which the model immediately begins using for inference.
- Mass customization: A model built with Orca can select from an unlimited number of different memorysets during inference. Since this process avoids retraining, even nuanced differences in the data (which typically gets lost or overfit during training) steer the model to make differentiated predictions for unique use cases. Your team also avoids the operational tax of having to maintain a proliferation of highly similar models.
LLMs can be used for classification and ranking, but they were not designed for non-generative use cases and have accuracy challenges, training/tuning limits, and speed/efficiency problems along with the well understood hallucination, forgetting, and context adherence problems. Orca’s memory driven architecture overcomes these limitations and combines the best of LLM retrieval augmentation with the better fit of ML models designed for these use cases.
Classifiers and rankers built on Orca does have several significant advantages over leveraging a generative model masquerading as a predictive AI:
- Higher accuracy: Purpose built models using the best available data typically have higher ceilings for their accuracy.
- Greater reliability: Because memories actively steer Orca’s predictive models, Orca’s models avoids risks of hallucinations or training data breakthroughs creating incorrect responses.
- Size & speed: Orca’s models tend to be smaller and more efficient. The size of a LLM - and the potential need for longer prompts - can create latency penalties that are painfully obvious to your users.
- Broader use cases: Orca supports use cases like image classification. LLMs only truly support natural language processing
Easiest way to get access to our platform is to email hello@orcadb.ai. If you’re up for it, share a few details about your specific system and what you’re hoping to unlock with Orca.
After this, you have several options for how you can test Orca:
- You provide a small, representative dataset (picture 3-5 examples per class) and we create a memory driven model to illustrate the core capabilities of Orca
- You provide a dataset for your use case and we create a complete Orca system to demonstrate technical and business value for your specific application.
- We collaboratively scope a proof-of-value project so you cannot only see the value of Orca’s system but develop capacity and understanding to operate and replicate it in your environment. You’ll have regular access to Orca engineers and resources to execute on a jointly developed plan with clear entry/exit criteria.
As experienced software developers, we know that adding new, hosted solutions into your tech stack can stress system performance in two critical ways - introducing noticeable, painful latency into your user experience and creating unworkable scaling challenges. Orca’s designed to manage both these challenges.
Latency: Making an API call to Orca and having Orca access external data sources during inference introduces the minor latency penalty that come with hosted solutions. However, this latency penalty — typically a few milliseconds — is generally very small and usually imperceptible to end users.
Orca can also improve latency compared to approaches injecting context through a re-purposed generative model. By shifting to a smaller, more efficient AIs that maintain specific context-aware memories, Orca may require less compute per input and a simpler, faster architecture.
Scaling: Orca has scaling characteristics very similar to a conventional database - meaning it can scale to nearly arbitrary read and write volumes, but you will at some point need to employ standard storage/database scalability techniques to get there (e.g., sharding, partitioning, read replicas, etc.)
Find out if Orca is right for you
Speak to our engineers to see if we can help you create more consistent models.